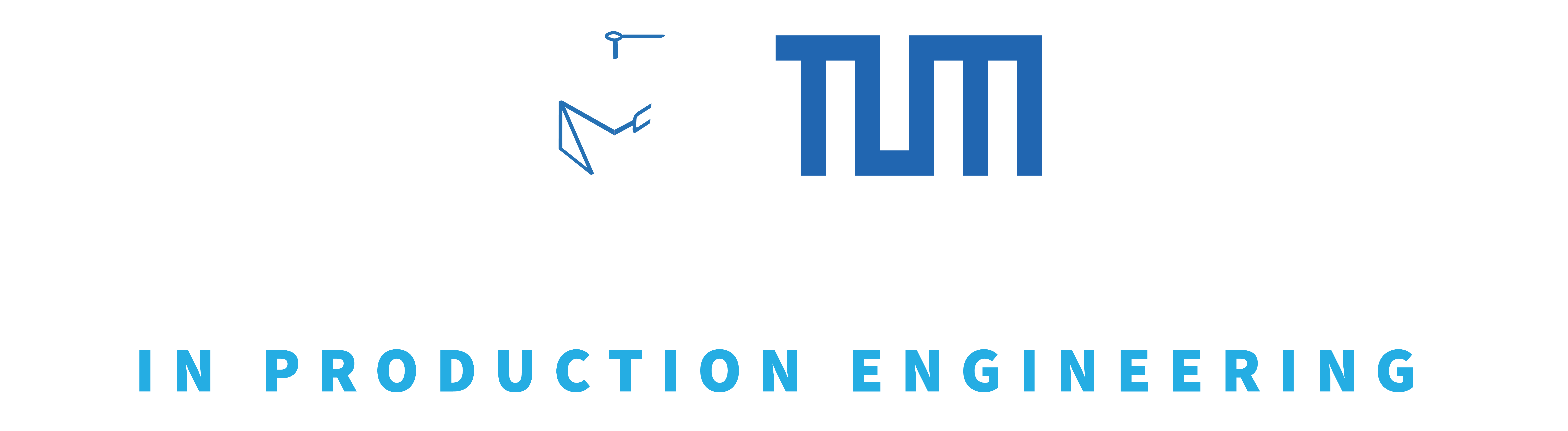
Designing safe, predictable, and high performance embedded platforms for next generation Cyber-Physical Systems
Led by Prof. Dr. Caccamo (Holder of Alexander von Humboldt Professorship in 2018), was founded in September 2018. We focus on designing safe, predictable, and high performance embedded platforms for next generation Cyber-Physical Systems, in which computing, communication, and control technologies are tightly integrated. Our target research areas are as follows: